The Holistic Innovation in Additive Manufacturing (HI-AM) Conference is the only academic conference in Canada focused exclusively on metal additive manufacturing. Over 100 additive manufacturing experts and decision-makers from academia, research institutes, industry and government participated in the 2021 event. At this same event, our team at Retinex presented our work in real-time geometry and solidification time prediction during laser additive manufacturing (LAM).
LAM is highly sensitive to small disturbances in the process inputs, resulting in variation in the geometry of the produced layer (clad). Since these changes are caused by complex metallurgical phenomena, it is difficult and computationally complex to derive a mathematical model of the entire process. To circumvent the modelling challenges, real-time monitoring can be implemented to measure geometry, and control methods to correct for any dimensional errors. The geometry monitoring is usually accomplished by processing the images captured by a camera. To extend the monitoring to thermal properties of the process (solidification time), an infrared camera is used. The addition of an infrared camera will further improve the accuracy of geometry measurement as the clad is observed in both cameras. The images are then input to a convolutional neural network to predict the geometry and solidification time for each timestep.
The images were captured using the MOC Sensor over the series of experiments shown in the image below.
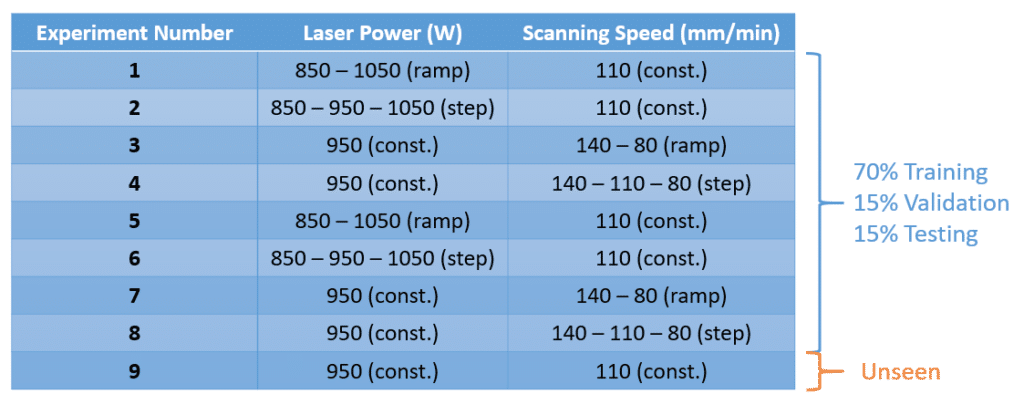
The first 8 experiments were used to train, validate and test a CNN with the architecture shown below. The CNN features convolutional and max pooling layers to decrease the size of the input thermal and vision images to determine the most valuable features. Once the images are reduced process parameters such as laser power and scanning speed are added to augment the data. The final layers of the CNN use this data to predict the clad width, clad height, and solidification time.
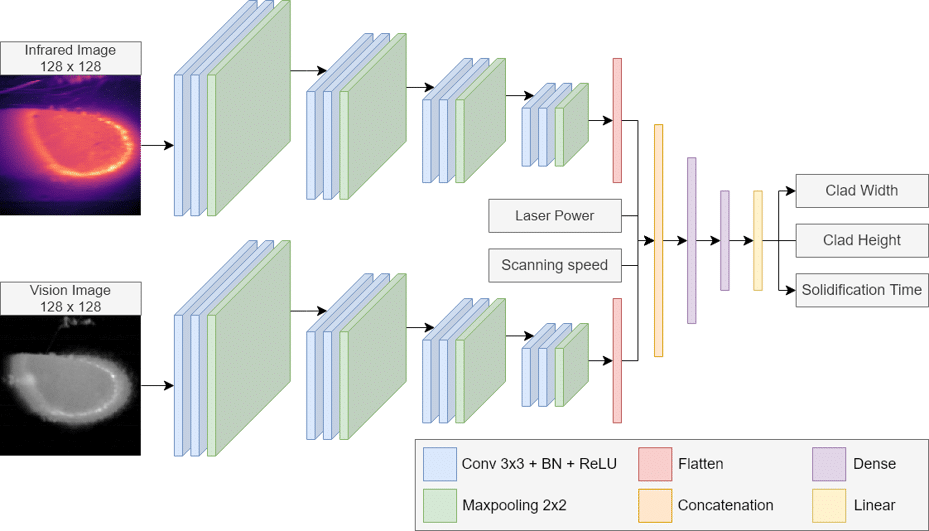
The performance of the trained CNN is summarized in the following image, which combines all of the first 8 experiments in one image. This dataset was shown to have a mean absolute percent error of less than 3.1% with an average computational time of approximately 0.045 seconds.
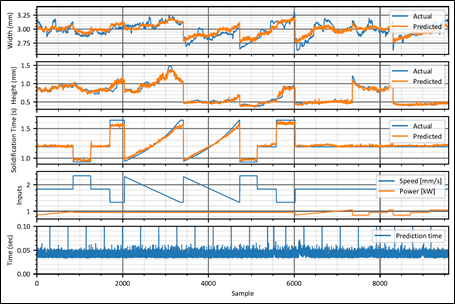
Finally, experiment 9 from the previous table was input to the CNN to determine the performance of data that was not used in training the CNN. The experiment was conducted with process settings that were within the scope of the other 8 experiments, but was not used in the training. Thus, this data represents the performance of the CNN against data not directly included in the training data.
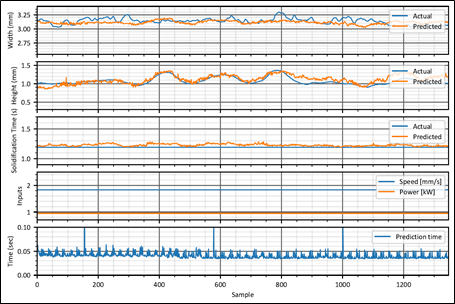
This data had a mean absolute percent error of less than 4% with an average computational time of approximately 0.045 seconds. Therefore, the CNN is shown to be sufficient in calculating the geometry and solidification of the melt pool during LAM accurately. Moreover, the computational time is within reasonable values such that this algorithm can be used for real-time process monitoring.